Killer robots are a staple of science fiction, but a recent letter by a group of more than 100 robotics experts, including founder of SpaceX Elon Musk, has warned the United Nations about the threat posed by lethal autonomous weapons, and requests that the use of artificial intelligence (AI) as a way to manage weaponry be added to the list of weapons banned by the UN Convention on Certain Conventional Weapons.
So why is now, when AI is being used for so much good, that they are so concerned about the threat it poses? To answer that we need to understand how we have arrived at where we are today and the rise of the conscious machines.
Intelligence gathering
Back in the summer of 1956, the fathers of artificial intelligence (AI) gathered at Dartmouth College, New Hampshire, to christen the new science and set its goals. Their concept of ‘human intelligence’ was quite narrow and specific. Computers would do what a rational, educated and mature man – for he was a man, and not a woman, that the fathers had in mind – did. He would use his knowledge and logic to solve complex problems. It was a goal that went beyond the purely numerical processing that computers were used for at that time.
The new science of artificial intelligence required a different computer that was capable of creating, storing and accessing knowledge, applying logical rules to facts and data, asking questions, concluding new facts, making decisions, and providing explanations for its reasoning and actions. Years before, the English mathematician Alan Turing had imagined an intelligent machine as one that would converse in our language and convince us of its human-ness. Nevertheless, the foundational machine intelligence aspirations had nothing to do with human feelings, morals, or consciousness. Although language understanding was included in the goals of early AI, the intention was not to replicate the human mind in a machine, but only mimic certain practical aspects of it. Besides, in the late 1950s our knowledge about the brain and mind was still in its infancy.
Read more about artificial intelligence:
- Hacking the economy to prosper in the coming age of artificial intelligence
- Far from being just a game, chess has always been a part of me
- Can humanity survive in the age of AI?
And yet, the temptation to think big was evident from the start. Already since 1943, pioneering neuroscientist Warren McCulloch and logician Walter Pitts had demonstrated the similarities between electronics and neurons. What if we could reproduce the whole human brain, with all of its intricate wiring, in an electronic computer? What if instead of describing to the computer how to think, we let it think by itself, and consequently evolve a ‘mind’ of its own? What if we made AI more human?
Then in 1956, the same year as the Dartmouth College Conference, US psychologist Frank Rosenblatt invented the ‘perceptron’, an algorithm that ran on specific neuron-mimicking hardware and was capable of learning similarly to a neural network: by strengthening or weakening the connections between neighbouring, interconnected neurons. The perceptron was the ancestor of artificial neural networks and deep learning, or what we today – 60 years later – understand as the big idea behind ‘artificial intelligence’.
Thinking like a human
The initial logical approach to AI produced some interesting results over the years, but ultimately ran into a dead end. Rosenblatt’s pioneering invention provided an alternative approach, one that enabled computers to go beyond logic, and venture into solving a really hard problem: perception. His work was almost forgotten for a while, but was resurrected by a new generation of brilliant scientists in the 1990s. With the cost of hardware capable of parallel processing dropping, it became possible to create algorithms that emulated the human brain. It was a technological breakthrough that redefined artificial intelligence and its goals.
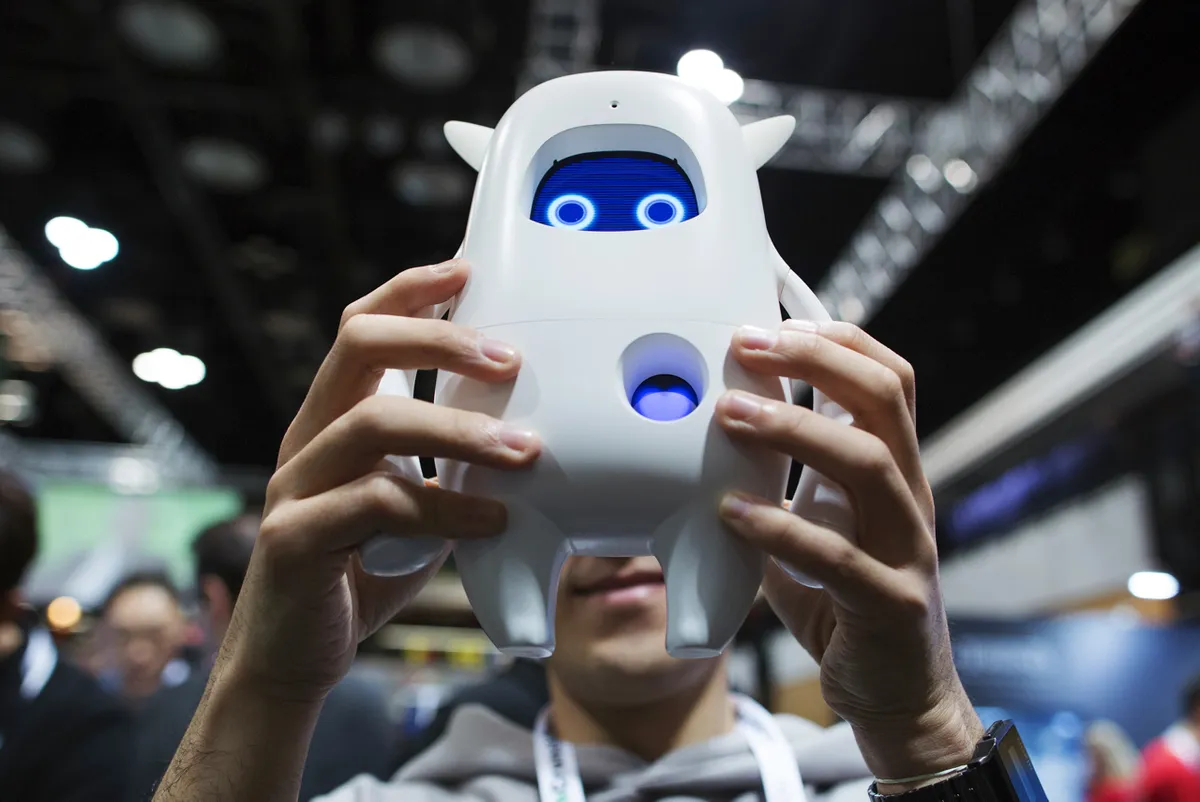
We now live in a time when intelligent machines are breaking records nearly every day. As billions of dollars of investment pour into AI research, machines are becoming smarter. The key to their accelerating smartness is their ability to learn. An artificial neural network – just like the ‘natural’ ones inside our brains – can learn to recognise facts by processing data through internal interconnections. For example, it can process the pixels of an image and recognise the face of a human, or an animal, or an object. And once artificial intelligence learns how to infer facts from data, it can do so again and again, much faster than our brains. Such machines need a lot of data in order to learn, and often a human trainer to supervise the learning. But machines can also learn by themselves, through a process called ‘reinforcement learning’. That was how AlphaGo, the algorithm developed by UK-based company DeepMind, was able to beat the world champion of Go. In a game famous for its complexity, the machine became an honorary 9-dan black belt master by playing against instances of itself.
AlphaGo was a watershed in the evolution of artificial intelligence because it offered a glimpse of the ultimate goal: the creation of general, human-like intelligence. To win at a complex game such as Go you need to think creatively, and use ‘intuition’. This means being able to draw from previous learnings and apply them effectively to new and unexpected problems. But computers are not there yet. They are still in the recognition intelligence phase: they can infer facts from data by recognising images, sounds, or human language, and make predictions based on their understanding of the data.
The next step towards becoming more ‘human’ requires machines to use their understanding in order to make real-time decisions and act autonomously. For example, it is not enough for a driverless car to recognise that a collection of pixels is a white van in front of it that is slowing down quickly. It must also reason that it needs to take evasive action. In doing so, it may have to decide between life and death. In other words, the next phase in AI evolution is for machines to enter the problematic areas of human morality.
The moral imperative for AI
The justification to make artificial intelligence more human-like is overwhelming. We value ‘intelligence’ as the cornerstone of our evolution. This value is deeply embedded in every human culture. What made our species survive against better-equipped predators was our ability to learn, invent and adapt. AI will turbocharge human intelligence and creativity. The economic reasons for pursuing ever-smarter machines are also profound.
Although artificial intelligence will disrupt many professions, such as manufacturing and retail, there is no other technology that has such potential to secure continuous economic growth and prosperity for future generations. For science, the advent of machines that can process vast amounts of data and discover new knowledge could not have come at a better time.
Listen to episodes of the Science Focus Podcast about AI:
- Why AI is not the enemy – Jim Al-Khalili
- Should we be worried about sex robots? – Kate Devlin
- What's the deal with algorithms? – Hannah Fry
Every scientific discipline is benefiting from AI to manage the data deluge. Physicists use it to research the fundamental laws of nature, biologists to discover new drugs for curing disease, doctors to provide better diagnoses and therapies. Pursuing the further development of machine intelligence for cultural, economic, and scientific reasons makes perfect sense. But as our machines become more human, and as more applications start to embed some artificial intelligence, a fundamental problem is beginning to emerge.
Emulating the human brain with artificial neural networks means that computers are mysterious and opaque. This is the so-called ‘black box problem’ of AI. In the brain and the machine, information is diffused in the network. When we retrieve a phone number from memory, we do not access a part of the brain where the number is somehow etched in flesh. Instead, each number is dispersed along multiple synapses that connect various neurons, at various levels of organisation. We do not really ‘know’ what we know, or how we know it. It is only because we possess consciousness that we are able to rationalise in retrospect, and thus ‘explain’ our intuitions and ideas.
As US neuroscientist David Eagleman has shown, most of what we become aware of has already happened in the brain at a non-conscious level. For humans, this has historically not been a problem, because we have assumed in our moral and legal systems that each of us is personally responsible for our thoughts and actions – at least when the chemistry of our brains is within socially-accepted ranges of ‘normality’. Nevertheless, for a non-conscious intelligent machine the ‘black box problem’ suggests that, although predictions and recommendations made by the machine may be accurate and useful, the machine will be incapable of explaining its reasoning. Imagine a driverless car taking a life and death decision, crashing, and killing a number of humans. With present day technology it is impossible to decode why the machine took the decision that it did.
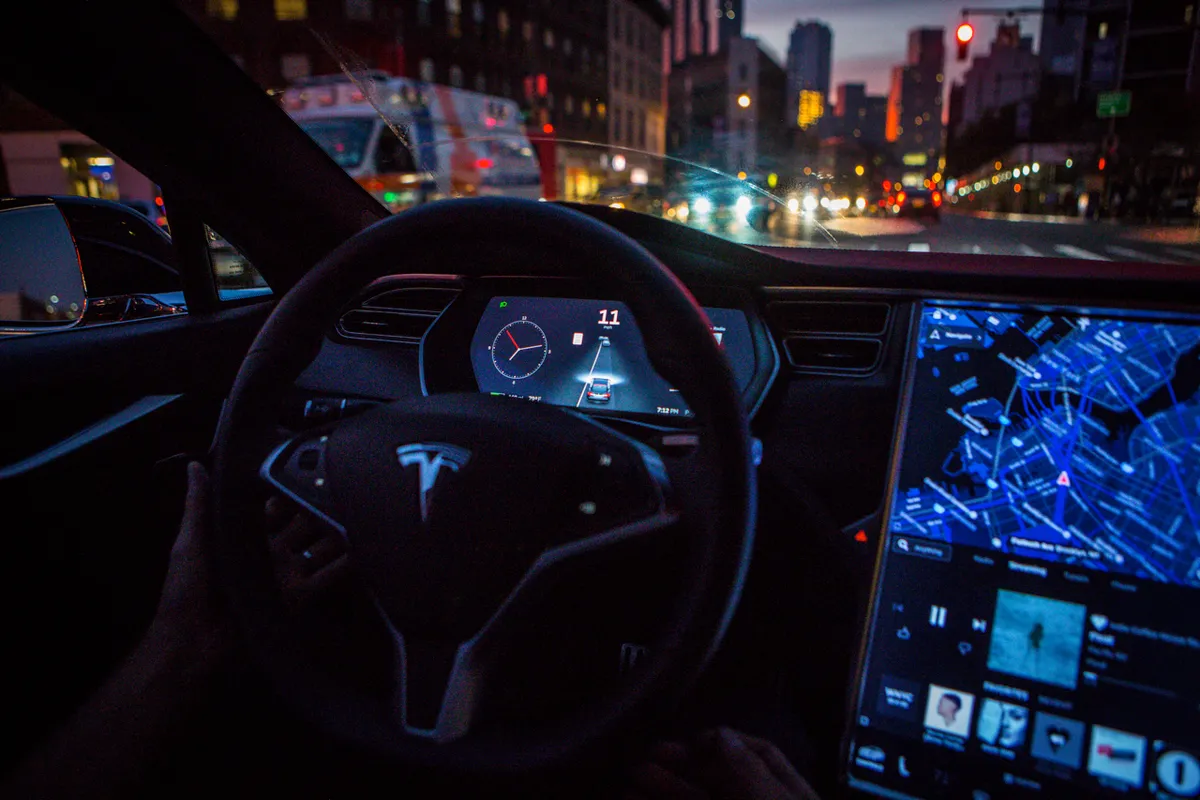
The black box problem is intensified when the data that a machine uses to learn has intrinsic biases, which could lead to biased conclusions or unsocial behaviour. In March 2016, for example, Microsoft released a bot on Twitter with the ability to learn human language by analysing live tweets. In less than 24 hours it had started tweeting racist and xenophobic rants. Scientists have been trying various approaches to solving the black box problem. In October 2016, DeepMind scientists published a paper in the journal Nature describing a ‘differentiable neural computing machine’ that combined a neural network with conventional external memory. Separating the processing from the data is a step towards ethically accountable intelligent machines. It makes it theoretically possible to code moral values that validate, or inhibit, the black box outcomes of neural networks. But this hybrid approach to developing safer artificial intelligence may not be enough in the future.
The real moral dilemma
After a bumpy start and much disappointment over the years, humanity has hit upon a technology with the potential to reshape everything. The economic and cultural impetus for exploiting the furthest boundaries of machine intelligence suggests that we will ultimately arrive at general, human-like intelligence, possibly in the next 10 to 20 years. Predictions may vary but if recent developments are a guide, we should expect general artificial intelligence sooner rather than later.
When this happens, we will have created machines capable of ingesting massive amounts of data and delivering superhuman insights and predictions. Ironically, and if the black box problem remains unsolved, we may then find ourselves in a position similar to the Ancient Greeks visiting the Oracle of Delphi and asking Apollo to predict their future. The language of the god was completely incomprehensible to humans so a human mediator – Pythia – was summoned to deliver cryptic utterances, which priests then interpreted in various, conflicting ways.
With artificial intelligence we are creating new gods whose intellect will far surpass our own. Their reasoning will be beyond our understanding. We will thus face an intolerable dilemma. Should we trust these new silicon gods on blind faith? It is very unlikely that human-level artificial intelligence with no ability to explain its reasoning will be socially acceptable. This leaves us with only one choice: to develop artificial intelligence even further, and equip it with human characteristics such as emotional intelligence, empathy and consciousness. Indeed, that would be the only way to solve the problem of communication between intelligent machines and us. The machines of the future will have to intuitively pick up our emotions and adapt to our moods and psychological profile. They will have to learn to tell how we feel, by analysing our voice and expressions, and drawing conclusions on the basis of the massive data they will have collected about us over the years. That is how they will be able to gain our trust and become part of human society.
Unlike our ‘human’ friends, these emotionally intelligent machines will know everything about us. We will not be able to hide anything from them. They will probably know much more about us than we would know about ourselves; and that’s how they will be able to guide us in making better decisions and choices in our lives. To have ‘someone’ like that in one’s life would be invaluable, and making artificial intelligence ever more human and empathetic would be welcomed.
Nevertheless, this dependency on such an intelligent machine poses a number of ethical questions. If we have a machine to always protect us from our errors, like a well-meaning and all-wise parent or partner, how will future humans learn from making mistakes, and therefore mature as individuals? Wouldn’t an all-caring, all-seeing AI result in the infantilisation of people and culture? And what about AI achieving consciousness? Should we be pushing the limits of technology to make machine self-aware? Would it be wise to breathe life into a lifeless jumble of wires, cooling fans and chips? Mary Shelley’s gothic masterpiece Frankenstein, and Ridley Scott’s Blade Runner provide useful insights to anyone aspiring towards such a future.
The limits of making artificial intelligence more human must surely be set before such a goal is ever achieved. Self-awareness will make machines capable of setting their own goals, which may be somewhat different from our own. Given our increasing dependency upon them in the future, those self-aware machines may well decide to manipulate our trust to meet their goals. And the master, who created an equal, may thus end up a slave.
This is an edited version ofThe Rise of the Conscious Machinesinissue 301ofBBC Focus magazine - for the latest science news, discoveries and innovations delivered to your doorsubscribe here.
Follow Science Focus onTwitter,Facebook, Instagramand Flipboard